April 29, 2024
Demystifying AI in Medicine: From Bootcamps to Bedside - A Deep Dive into the Mount Sinai Workshop
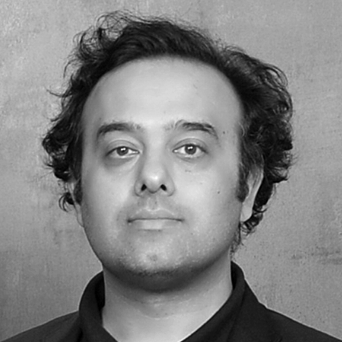
Ali Madad
Author
Demystifying AI in Medicine: From Bootcamps to Bedside - A Deep Dive into the Mount Sinai Workshop
The AI in Medicine workshop at Mount Sinai, a groundbreaking initiative spearheaded by medical students Jen (Medical Student, Mount Sinai), Katie (Medical Student, Mount Sinai), and Joy Chen (MD-PhD Candidate, Mount Sinai), was far more than just an introductory session. It was a deep, immersive exploration into a rapidly transforming field, a "bootcamp," as organizer Jen described it, meticulously designed to equip attendees, regardless of their prior technical expertise, with the foundational knowledge and critical perspectives essential for navigating the complex and often bewildering world of artificial intelligence in healthcare.
This "super casual workshop," as Jen noted, was envisioned as "the first of many workshops to come over the years," and it delivered on its promise to foster a shared understanding and spark crucial conversations about AI's role in shaping the future of medicine.
Spanning two days, the workshop provided a holistic view, moving beyond the hype to grapple with the practical realities, ethical dilemmas, and transformative potential of AI.
Through presentations, interactive sessions, and case studies, a diverse faculty comprised of fellow medical students and renowned physician-scientists created a uniquely enriching learning environment, fostering a collaborative spirit and encouraging open dialogue.
Thematic Insights from the Workshop: A Multifaceted Exploration of AI in Medicine
Rather than simply recounting the workshop chronologically, this summary delves into the key thematic threads that wove through the presentations and discussions, offering a more integrated and insightful understanding of the multifaceted landscape of AI in medicine.
1. The State of the Art: AI's Expanding Footprint Across Medical Modalities
The workshop provided a compelling panorama of state-of-the-art AI applications, demonstrating its growing reach and influence across diverse medical specialties and modalities.
Speakers showcased AI's capacity to augment human capabilities, improve diagnostic accuracy, and streamline clinical workflows in ways previously unimaginable.
Natural Language Processing (NLP): Unlocking Insights from the Language of Medicine
Anish Kumar, a Mount Sinai medical student, expertly debunked the common misconception that NLP is synonymous with chatbots.
He asserted, "NLP does not equal chatbots," emphasizing the field's rich history and broad scope, stating, "NLP is a field that's natural language processing... It's become extremely visible... because of chat GPT and the sort of burgeoning use of LLMs... But this field has the decades long history going back all the way to like World War II."
Kumar illuminated NLP's diverse applications, highlighting its ability to perform "sentiment analysis" on patient feedback, enabling healthcare providers to gauge patient experiences and improve care delivery.
He further showcased NLP's power in "information extraction" from the vast amounts of unstructured text within EHRs, potentially automating tasks like chart review and identifying key clinical insights buried within narrative notes.
Machine translation, another key application, promises to break down language barriers in healthcare, improving communication and access for diverse patient populations.
Kumar emphasized that NLP is fundamentally about enabling machines to "process, manipulate, interpret, and act upon information from human languages," a capability with profound implications for a field so heavily reliant on language and communication.
Computer Vision: Seeing Beyond the Human Eye in Medical Imaging
The workshop resounded with the transformative impact of computer vision and deep learning in revolutionizing medical imaging.
Felix Richter, in his captivating case study on AI in the NICU, demonstrated how computer vision, specifically "pose estimation," can objectively quantify subtle, yet clinically critical, cues like infant alertness from video data – a feat beyond the capabilities of human observation alone.
Dr. Alisa Ruch further showcased the cutting edge of AI in cardiology imaging, presenting AI models capable of "automated echocardiogram interpretation," even "outperforming human experts in challenging tasks like mitral regurgitation assessment."
Dr. Ruch highlighted a groundbreaking AI model for chest X-ray analysis that, by learning from echocardiogram labels, could "detect severe LVH and dilated left ventricles" and surpass the diagnostic accuracy of "15 radiologists in a head-to-head comparison."
This demonstrated AI's potential not just to automate image interpretation but to enhance diagnostic accuracy by extracting previously imperceptible information from medical images, pushing the boundaries of what's clinically discernible.
The "hallucination" techniques in PET scans, as Dr. Ruch noted, further illustrate AI's ability to enhance image quality and potentially reduce radiation exposure, showcasing AI's role in making medical imaging safer and more efficient.
Reinforcement Learning: Personalizing and Adapting Healthcare in Real-Time
Joy Chen and Corbin Matthews introduced the audience to the exciting frontier of Reinforcement Learning (RL) and its unique suitability for medicine.
They explained that RL empowers AI systems to learn through trial-and-error and feedback, enabling them to adapt treatment strategies dynamically to individual patient needs.
Corbin Matthews illustrated this with the example of "optimizing CPAP pressure for patients with respiratory disease" and "optimizing the timing and dosage of let's say hypertension or diabetic medications for a patient based on prior states of the patient," emphasizing RL's promise for truly "personalized and adaptive health care interventions in complex and dynamic settings."
This capacity for real-time learning and adaptation distinguishes RL from other ML paradigms and positions it as a powerful tool for managing the complexities and variability inherent in patient care.
Democratizing AI Innovation: The Power of No-Code/Low-Code Platforms
Vivian's interactive workshop offered a refreshing and empowering perspective by showcasing the accessibility of AI development through no-code/low-code platforms.
Challenging the notion that AI is solely the domain of expert programmers, Vivian’s session demonstrated that "no cold machine learning is really important because it... essentially makes ML more accessible, particularly to a wider range of people across like healthcare, finance, pretty much any industry."
By guiding participants through the creation of a chest X-ray classifier using Google Cloud AutoML, Vivian demystified the AI building process, proving that clinicians and researchers without extensive coding skills can actively participate in AI innovation.
This democratization of AI development holds immense potential for fostering wider adoption and ensuring that AI solutions are truly tailored to the needs and insights of those on the front lines of healthcare.
2. Navigating the "Hope vs. Hype" Dichotomy: Unveiling Open Questions and Research Frontiers
While celebrating the remarkable advancements in AI, the workshop also maintained a critical and balanced perspective, carefully navigating the "hope vs. hype" landscape.
Speakers consistently emphasized the open questions, challenges, and ongoing research needed to ensure responsible and impactful AI implementation in medicine.
The Evidence Gap: The Urgent Need for Clinical Validation
Dr. Gaurishan (Ned) Karni, in his keynote address, cautioned against the "tipping block between hope and hype," emphasizing the critical "lack of robust evidence from randomized controlled trials" to validate the real-world clinical impact of AI interventions.
He pointed to the "BMJ paper in 2020, looking at COVID models," where a staggering "90% of them had high risk of bias and were not generalizable at all," underscoring the potential dangers of deploying unvalidated AI models in clinical settings.
Dr. Ruch further emphasized this point, highlighting the "CACTIS" trial as a crucial example of prospective validation, aiming to assess the real-world impact of AI safety nets in emergency departments.
This collective emphasis on rigorous clinical validation signaled a crucial shift in the field, moving beyond proof-of-concept studies to demanding robust evidence of clinical benefit and patient safety.
Addressing Data's Inherent Flaws and Biases: A Multifaceted Challenge
The workshop speakers collectively acknowledged and grappled with the inherent limitations and biases embedded within healthcare data.
Divya dedicated her entire presentation to "all the data we cannot see," meticulously outlining how factors like "missing data, biased data collection, misdiagnosis, and limited representation" can skew datasets and undermine the fairness and reliability of AI models.
Dr. Lily Chan's research on "stigmatizing language in EHRs" provided a stark example of how deeply ingrained biases can manifest within clinical text data, potentially perpetuating health disparities.
Akil Merchant, in his practical guide to ML experiments, underscored the time-consuming but essential task of "data collection, cleaning, pre-processing, and understanding its limitations" as the bedrock of any responsible AI project.
These discussions collectively highlighted that addressing data flaws and biases is not a mere technical hurdle but a fundamental ethical and scientific imperative for building trustworthy and equitable AI systems in healthcare.
The Black Box Paradox: Transparency, Interpretability, and Trust
While celebrating the power of complex AI models, speakers also confronted the persistent challenge of interpretability and transparency.
Anish Kumar raised the pertinent ethical question of "transparency" in AI-driven patient interactions, particularly with chatbots, questioning, "How transparent do you think we have to be to patients like when that the efficacy of the tool might hinge on the fact that it's coming from a human agent?"
Dr. Karni, while acknowledging the value of interpretability, sparked a thought-provoking debate by questioning its absolute necessity, suggesting that "the ultimate thing is whether you're going to believe this recommendation or not."
He argued that while transparency is desirable, the focus should ultimately be on building trust in AI systems, even if their inner workings remain somewhat opaque.
Akil Merchant's detailed explanation of neural networks aimed to demystify these "black boxes," emphasizing that understanding the underlying mathematical principles and evaluation metrics is crucial for building informed trust in AI recommendations.
Calibration, Uncertainty, and the Nuances of Clinical Decision-Making
Akil Merchant's presentation underscored that AI models, at their core, provide probabilistic outputs, not definitive yes/no answers.
He stressed the critical importance of "calibration" to ensure that these probabilities are clinically meaningful and accurately reflect real-world risks.
He challenged the audience to grapple with the nuances of clinical decision-making in the face of probabilistic AI recommendations, asking, "What's the difference between 47% and 51%? It's a purely academic thing at that point."
This highlighted the need for clinicians to develop new frameworks for integrating AI-driven probabilities into their clinical judgment, moving beyond simplistic binary interpretations and embracing the inherent uncertainties of medical prediction.
3. Charting the Future: Responsible AI, Clinician Empowerment, and a Human-Centered Approach
The workshop concluded with a powerful call to action, emphasizing the need for responsible AI development, clinician empowerment, and a human-centered approach to guide the future of AI in medicine.
The Imperative of Responsible AI
Katie's presentation on "Responsible AI" and Divya's work on addressing data limitations served as cornerstones for this concluding theme.
Katie outlined the core principles of "reliability, fairness, safety, transparency, privacy, consent, and accountability," providing a clear ethical compass for navigating the complex landscape of AI in healthcare.
Divya's research further underscored the ethical imperative of addressing bias and promoting equity, arguing for the use of "granular race data" and methods like "PRPL" to mitigate underreporting bias and ensure fairer algorithmic outcomes.
This collective emphasis on responsible AI signaled a commitment to developing AI technologies that are not only powerful but also ethical, equitable, and aligned with the best interests of patients.
Empowering Clinicians: Shaping the AI Revolution from the Inside
Speakers consistently emphasized that AI is not intended to replace clinicians but to augment and empower them.
Dr. Karni envisioned a future where AI "will help us become better physicians," freeing up time for "more meaningful sort of physician-patient interaction."
Katie highlighted the diverse and evolving roles for clinicians in the AI era, encouraging medical professionals to become "informed users," "educators," "clinical collaborators," "innovators," and "advocates" for responsible AI implementation.
Vivian’s no-code workshop directly empowered clinicians to become active participants in AI development, breaking down technical barriers and fostering a sense of ownership and agency in shaping the future of AI in their field.
Felix Richter’s personal journey, transitioning from PhD-level AI research to clinical practice in the NICU, exemplified the power of clinician-led AI innovation, demonstrating how firsthand clinical experience can drive the development of truly impactful AI solutions.
A Human-Centered Approach: Keeping Compassion and Human Values at the Forefront
Despite the focus on advanced technologies, Dr. Karni’s concluding remarks served as a powerful reminder that the "magic ingredients to improving patient outcomes in this AI age will be the same as they have been in the history of medicine and the history of humankind."
He underscored the enduring importance of "rigor, compassion, imagination, thoughtful leadership, multidisciplinary teamwork, and a very healthy dose of luck," reminding attendees that technology, however powerful, must always be guided by human values and a deep commitment to patient well-being.
This human-centered approach, emphasizing compassion, ethical considerations, and the unique strengths of human clinicians, emerged as the ultimate guiding principle for navigating the AI revolution in medicine.
Conclusion: Moving Forward Responsibly and Collaboratively
The AI in Medicine workshop at Mount Sinai was not merely a technical overview but a thoughtful and nuanced exploration of a transformative force in healthcare.
By demystifying complex AI concepts, showcasing state-of-the-art applications, and confronting the critical challenges and ethical dilemmas, the workshop provided a crucial roadmap for navigating the future of AI in medicine responsibly and collaboratively.
The emphasis on data quality, bias mitigation, transparency, clinician empowerment, and a human-centered approach underscored the need for a multi-faceted strategy, one that combines technological innovation with ethical foresight and a deep commitment to improving patient care for all.
As the field continues to evolve at an unprecedented pace, the lessons and conversations sparked at this workshop will undoubtedly serve as a valuable guide for clinicians, researchers, and policymakers alike, ensuring that the AI revolution in medicine is one that truly benefits humanity.
Get in Touch
Want to learn more about how we can help your organization navigate the AI-native era?