March 10, 2025
AI Engineering Conference: AI Leadership & The Path to Real-World Impact
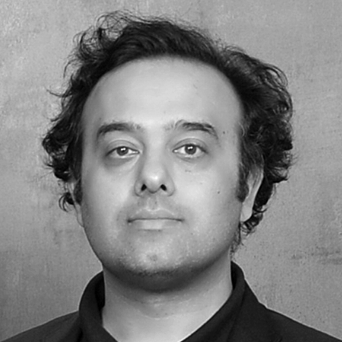
Ali Madad
Author
The first day of the AI Engineering Conference set the stage for a new kind of AI leadership—one focused not just on technological advancement, but on the organizational and strategic shifts needed to make AI work at scale.
Speakers from across the AI landscape explored how companies can move beyond experimentation and into real-world AI deployment.
The message was clear: AI isn't just a technical problem—it's a leadership challenge.
High-Level Themes: AI Leadership as Organizational Transformation
The day revolved around five key themes:
- From Experimentation to Execution – AI leaders must shift from endless pilots to structured, measurable execution.
- AI as a System, Not a Feature – Organizations must rethink workflows and processes around AI rather than adding AI to existing ones.
- Measuring AI Success the Right Way – Businesses need to move beyond accuracy metrics and focus on real business impact.
- Building the Right AI Infrastructure – AI isn't just about models; observability, governance, and scaling systems are just as critical.
- AI & The Human Workforce – AI is shifting jobs, not just automating them—upskilling and AI literacy are now competitive advantages.
"Your AI roadmap is your business roadmap. If it's not, you don't have an AI strategy—you have a science project."
Speaker Insights: The Challenges & Opportunities of AI at Scale
AI in the Enterprise: Breaking Through the Hierarchy
@steveonjava of @neo4j & Jonathan Lowe of @pfizer
"It's really about that business value and being able to partner with the different parts of the organization because, you know, R&D is very different than commercial."
For AI to succeed, it must navigate complex corporate structures, bridging the gap between research, product, and business objectives.
AI Agents & Workflow Automation
Shirsha Ray Chaudhuri of @thomsonreuters
"We are now here where we are looking at automating entire workflows with the use of AI/agents, and not just one task at a time."
The shift isn't just about AI-powered tools, but about reimagining how work gets done—integrating multi-agent AI to handle complex, multi-step processes.
AI Observability: Don't Let Black Boxes Run Your Business
@diamondbishop of @datadoghq
"Observability is actually really important. And don't make it an afterthought. These are complex workflows. You really need situational awareness to debug problems."
AI systems are only as good as their debugging and tracking mechanisms. Without observability, failures can go unnoticed until they become catastrophic bottlenecks.
@aparnadhinak of @arizeai
"Evals aren't just at one layer of your entire trace... The goal here is really, how do you make sure that you have evals throughout your application, so that when something goes wrong, I can debug if it actually happened at the router level, if it happened at the skill level, or if it happens somewhere else along the flow?"
RAG & AI in Production: Harder Than It Looks
@douwekiela of @ContextualAI
"Pilots are very easy... But getting this to work at tens of thousands... It's very, very difficult to do that."
Many companies underestimate the gap between a working AI demo and full-scale production deployment.
Data Governance: Not Just Compliance—A Competitive Advantage
@BoscoDurai of @privacera
"Data Governance isn't just about avoiding risk, it's about accelerating reward. By proactively governing data, you empower your organization to move faster and more confidently with AI, turning data from a potential liability into a true strategic asset."
Organizations that treat data governance as a growth enabler, rather than just a compliance necessity, will outpace competitors in AI adoption.
AI Infrastructure: It's Scaling Faster Than You Think
Paul Gilbert of @AristaNetworks
"Things are moving fast. We're at 800 gig right now, which we have been for probably a year. We will see 1.6 terabytes on the network probably end of this year, early 2027. And it will just keep growing and growing and growing."
The hardware and infrastructure layer of AI is often overlooked, yet it's one of the biggest constraints on AI scaling.
The Agent Dynamic: Compounding Gains & Cumulative Errors
AI agents are powerful, but they come with new risks and challenges.
@graceisford of @Lux_Capital
"Cumulative errors add up."
@colin__flaherty of @augmentcode
"I think the exciting thing about AI and code is this idea of compounding gains."
AI's strengths—automation, continuous learning, and self-improvement—can either accelerate business performance or amplify existing flaws if not carefully managed.
Building the Foundation: Platforms, Talent & AI Evolution
AI Platforms: Empowering Teams, Not Just Automating Work
@brunopassos of @bookingcom & @beyang of @Sourcegraph
"We believe that by building a platform, we can empower all the product teams at Booking.com to leverage the power of generative AI."
AI Talent: Experience Now Trumps Credentials
@heathwblack of @signalfire
"Work experience has always been important, but it now far surpasses education in terms of the main aspect that you should be looking at here."
Companies need to rethink hiring—prioritizing hands-on AI experience over traditional credentials like PhDs.
AI & Customer Success: Solving the Real Problem
@ambricken & Joe Bayley of @AnthropicAI
Joe Bayley:
"How can they use AI to solve the core problem that your product is trying to solve?"
The best AI companies aren't just deploying technology—they're aligning AI to solve their customers' actual pain points.
What Comes Next? The Transition from Leadership to Execution
Day 1 of the AI Engineering Conference made one thing clear:
AI Leadership is about transformation—technological, organizational, and strategic.
But execution matters.
As the conference moves into Day 2: AI Engineering & Implementation, the focus will shift from high-level strategy to practical AI deployment.
Organizations that succeed won't just have better AI models—they will have:
- AI-native workflows
- Scalable infrastructure
- Clear AI evaluation & observability
- A workforce trained to work with AI
The companies that get this right will lead the AI era. Everyone else will be playing catch-up.
Get in Touch
Want to learn more about how we can help your organization navigate the AI-native era?